《The effect of spatial resolution on decoding accuracy in fMRI multivariate pattern analysis》
一、简介
论文的基本信息
标题: The effect of spatial resolution on decoding accuracy in fMRI multivariate
pattern analysis
作者:
Anna Gardumi a,b,
⁎, Dimo Ivanov a,b
, Lars Hausfeld a,b
, Giancarlo Valente a,b
, Elia Formisano a,b
, Kâmil Uludağ a,b
机构:
a Department of Cognitive Neuroscience, Faculty of Psychology and Neuroscience, Maastricht University, Maastricht, The Netherlands
b Maastricht Brain Imaging Center, Maastricht University, Maastricht, The Netherlands
摘要
fMRI中的多变量模式分析(MVPA)已被用于从分布的皮层激活模式中提取信息,这在传统的单变量分析中可能无法检测到。然而,对于fMRI中MVPA的物理和生理基础以及空间平滑对其性能的影响知之甚少。一些研究已经解决了这些问题,但他们的调查仅限于3岁时的视觉皮层,结果相互矛盾。在这里,我们使用超高场(7 T)功能磁共振成像来研究空间分辨率和平滑对语音内容(元音)解码和说话者身份的影响。为此,我们获得了高分辨率(1.1 mm各向同性)的fMRI数据,并从原始k空间数据中以2.2和3.3 mm的平面内空间分辨率重建了它们。此外,每个分辨率下的数据都用不同的三维高斯核尺寸进行空间平滑(即不平滑或1.1、2.2、3.3、4.4或8.8 mm核)。对于所有空间分辨率和平滑核,我们证明了使用支持向量机(SVM) MVPA在7 T下解码语音内容(元音)和说话人身份的可行性。此外,我们发现高空间频率对元音解码具有重要的信息作用,并且在两种解码任务中,高空间频率和低空间频率的相对贡献是不同的。适度平滑(高达2.2 mm)提高了元音和扬声器解码的精度,可能是由于减少了噪声(例如残余运动伪影或仪器噪声),同时仍然保留了高空间频率的信息。总之,我们的结果表明,即使在相同的刺激和相同的大脑区域,fMRI中MVPA的最佳空间分辨率取决于感兴趣的特定解码任务。
Multivariate pattern analysis (MVPA) in fMRI has been used to extract information from distributed cortical activation patterns, which may go undetected in conventional univariate analysis. However, little is known about the physical and physiological underpinnings of MVPA in fMRI as well as about the effect of spatial smoothing on its performance. Several studies have addressed these issues, but their investigation was limited to the visual cortex at 3 T with conflicting results. Here, we used ultra-high field (7 T) fMRI to investigate the effect of spatial resolution and smoothing on decoding of speech content (vowels) and speaker identity from auditory cortical responses. To that end, we acquired high-resolution (1.1 mm isotropic) fMRI data and additionally reconstructed them at 2.2 and 3.3 mm in-plane spatial resolutions from the original k-space data. Furthermore, the data at each resolution were spatially smoothed with different 3D Gaussian kernel sizes (i.e. no smoothing or 1.1, 2.2, 3.3, 4.4, or 8.8 mm kernels). For all spatial resolutions and smoothing kernels, we demonstrate the feasibility of decoding speech content (vowel) and speaker identity at 7 T using support vector machine (SVM) MVPA. In addition, we found that high spatial frequencies are informative for vowel decoding and that the relative contribution of high and low spatial frequencies is different across the two decoding tasks. Moderate smoothing (up to 2.2 mm) improved the accuracies for both decoding of vowels and speakers, possibly due to reduction of noise (e.g. residual motion artifacts or instrument noise) while still preserving information at high spatial frequency. In summary, our results show that – even with the same stimuli and within the same brain areas – the optimal spatial resolution for MVPA in fMRI depends on the specific decoding task of interest.
二、论文主要内容
语音刺激的解码任务
作者使用了一个实验范式和一组刺激,这些刺激在之前的3 T fMRI研究中被使用过(Formisano et al., 2008)。他们呈现了不同说话者的语音刺激(元音),并考虑了从听觉皮层反应模式中解码元音和说话者的单试验。
本文使用了超高场(7T)fMRI技术,从听觉皮层的反应模式中对语音刺激(元音)和说话者进行单次解码。具体来说,本文采用了以下步骤:
- 刺激设计:本文使用了不同说话者发出的不同元音作为刺激,共有六个元音(/a/, /e/, /i/, /o/, /u/, /y/)和六个说话者(三男三女)。
- 数据采集:本文在7T扫描仪上采集了高分辨率(1.1 mm等距)的fMRI数据,并3. 从原始k空间数据重建了不同的有效空间分辨率(2.2 mm和3.3 mm)的数据。
数据处理:本文对每种空间分辨率的数据进行了不同程度的空间平滑处理(无平滑或1.1, 2.2, 3.3, 4.4, 8.8 mm的高斯核)。 - 数据分析:本文使用支持向量机(SVM)MVPA方法,对每种空间分辨率和平滑条件下的数据进行了元音和说话者的解码分析,并比较了不同条件下的解码准确率。
多变量模式分析(MVPA)
多变量模式分析中的多变量指的是多个变量之间的关系。它是一种基于机器学习的方法,用于从fMRI数据中提取空间模式信息,并用这些信息来解码不同的认知过程。多变量模式分析可以同时考虑多个脑区的活动模式,从而提高解码的准确性和稳定性。它是一种相对于单变量分析和双变量分析更加复杂和全面的数据分析方法。
多变量模式分析(MVPA)是一种用于从fMRI数据中提取空间分布的激活模式的信息的方法。
这是一种用于从功能磁共振成像(fMRI)数据中提取空间分布的激活模式的信息的方法,它可能在传统的单变量分析中无法检测到。
本文的MVPA主要包括以下几个方面:
- 实验范式和刺激:作者使用了7T的fMRI设备,采集了高分辨率(1.1 mm等距)的fMRI数据,并从原始k空间数据重建了不同的有效空间分辨率(2.2 mm和3.3 mm)。作者使用了不同发音者的元音作为语音刺激,并考虑了从听觉皮层反应模式中解码元音和发音者的单试验。
- 解码方法和评估指标:作者使用了支持向量机(SVM)作为MVPA的解码方法,使用了准确率(accuracy)作为评估指标。准确率是指正确预测的试验数占总试验数的比例。作者对每个空间分辨率和每个平滑核大小的数据进行了十次交叉验证,并计算了平均准确率和标准差。
- 结果和结论:**(1)作者发现,对于所有的空间分辨率和平滑核大小,都可以从听觉皮层的fMRI数据中解码出元音和发音者的信息。(2)此外,作者发现,高空间频率的信息对于元音解码是有用的,(3)而不同的解码任务对于不同空间频率的信息的贡献是不同的。(4)适度的平滑(最多2.2 mm)可以提高元音和发音者的解码准确率,可能是由于降低了噪声(例如残余运动伪影或仪器噪声),同时仍然保留了高空间频率的信息。(5)**总之,作者的结果表明,即使在相同的刺激和相同的脑区的情况下,fMRI数据的MVPA的最佳空间分辨率也取决于感兴趣的具体解码任务。
K空间
在磁共振成像(MRI)中,k空间或频率空间是通过测量的图像的二维或三维傅里叶变换得到的。k空间中的每个点都代表了一个特定的空间频率,即图像中不同空间尺度的变化程度。k空间的坐标轴对应于图像的x和y轴,而k空间的坐标值对应于图像中不同空间频率的分量。因此,k空间可以看作是一个空间频率域,其中每个点代表了一个特定的空间频率分量。
在本文中,k空间数据是指从MRI扫描中收集的原始数据,其中每个点代表了一个特定的空间频率分量。重建过程是将k空间数据转换为图像数据的过程。具体来说,重建过程涉及将k空间数据进行傅里叶变换,以获得图像的空间域表示。在重建过程中,可以使用不同的算法和滤波器来处理k空间数据,以获得不同的图像质量和空间分辨率。在本文中,作者使用了不同的3D高斯核大小对每种空间分辨率的数据进行了空间平滑处理,以评估空间分辨率对MVPA解码性能的影响2。
具体来说:
k空间的数值是指k空间中每个点的坐标值,它们表示了图像中不同空间频率的分量。空间频率是指图像中不同空间尺度的变化程度,比如图像中的细节、边缘、对比度等。k空间中的数值越大,表示空间频率越高,图像中的变化越快,细节越丰富。k空间中的数值越小,表示空间频率越低,图像中的变化越慢,细节越少。
k空间的量纲是长度的倒数,即1/米。这是因为k空间中的坐标值是由波矢k决定的,而波矢k的定义是k=2π/λ,其中λ是波长,单位是米。因此,k的单位是1/米,k空间的量纲也是1/米。k空间的量纲和实空间的量纲是互为倒数的关系,这也反映了傅里叶变换的性质。
根据本文,MVPA中的多变量是指不同的声音刺激(元音和说话者)以及不同的空间分辨率和平滑核。这些变量影响了fMRI数据中的激活模式,从而可以用于解码声音信息。本文的目的是研究不同的空间分辨率对MVPA解码性能的影响。
空间分辨率和平滑对MVPA的影响
作者使用超高场(7 T)fMRI采集了高分辨率(1.1 mm等距)的数据,并从原始k空间数据重建了不同的有效空间分辨率,以评估空间分辨率对MVPA解码性能的影响。他们还对每种分辨率的数据进行了不同的3D高斯核大小的空间平滑处理。
平滑的具体过程
- 作者使用了不同的3D高斯核大小(即无平滑或1.1、2.2、3.3、4.4或8.8 mm核)对每种空间分辨率的数据进行了空间平滑处理。
- 空间平滑处理是一种图像处理技术,它可以减少图像中的噪声和细节,使图像更加平滑和模糊。
- 本文中的空间指的是fMRI数据的三维空间,即沿着x、y和z轴的方向。空间平滑处理会影响fMRI数据中的空间频率,即图像中不同空间尺度的变化程度。
流程详解
流程图
这个流程图主要讲了如何用不同的空间分辨率重建fMRI数据的方法。它包括以下几个步骤:
原始数据采集:用7T的fMRI扫描仪采集高分辨率(1.1 mm 立方体)的fMRI数据,同时记录k-空间数据。
数据重建:用不同的采样因子(1, 2, 3)从原始的k-空间数据中提取不同的分辨率(1.1, 2.2, 3.3 mm 平面)的fMRI数据。
数据平滑:用不同的高斯核(0, 1.1, 2.2, 3.3, 4.4, 8.8 mm 立方体)对每个分辨率的fMRI数据进行空间平滑处理。
数据分析:用支持向量机(SVM)多变量模式分析(MVPA)对每个分辨率和平滑条件下的fMRI数据进行语音内容(元音)和说话者身份的解码。
Original high resolution data
magnitude 图像是由复数 MRI 信号的两个分量分别表示的。phase 图像反映了信号的相位信息,magnitude 图像反映了信号的幅度信息。phase 图像可以用来显示血流和磁化率引起的失真,magnitude 图像可以用来显示组织的密度和对比度。
两个图像有以下区别:
phase 图像的分辨率更低,因为相位信息受到空间分辨率的影响。相位图像中的跳变或不连续性会导致幅度图像中的伪影。
magnitude 图像的分辨率更高,因为幅度信息受到信噪比的影响。幅度图像中的细节和边缘信息更清晰,可以用来识别不同的组织和结构。
2D FFT
2D FFT方法是指二维快速傅里叶变换(Fast Fourier Transform)方法,它是一种用于将二维信号从时域转换到频域的数学算法。它可以将一个二维矩阵(如图像)分解成一组正弦和余弦函数的加权和,从而得到该矩阵在频域上的表示。2D FFT方法在图像处理、信号处理、通信等领域有着广泛的应用,可以用来提取图像的频域特征,进行图像滤波、增强、压缩等操作。
Downsampling of the k-space
在本文中,Cropping和zero–padding是两种用于改变fMRI数据空间分辨率的方法。Cropping是指从原始k-space数据中截取一部分数据,然后进行重建,从而得到更高分辨率的图像。Zero-padding是指在原始k-space数据的边缘添加零值,然后进行重建,从而得到更低分辨率的图像。这两种方法都可以用来探索不同空间分辨率对MVPA解码性能的影响。本文的作者使用了这两种方法,将1.1 mm等距的高分辨率数据重建为2.2 mm和3.3 mm的不同分辨率,并对每种分辨率的数据进行了不同程度的空间平滑处理,以评估空间分辨率和平滑核大小对解码准确率的影响。
2D IFFT
2D IFFT是指二维离散傅里叶逆变换(Inverse Fast Fourier Transform),它是一种用于将二维信号从频域转换到时域的数学算法。它可以将一个二维矩阵(如频域图像)分解成一组正弦和余弦函数的加权和,从而得到该矩阵在时域上的表示。2D IFFT方法在图像处理、信号处理、通信等领域有着广泛的应用,可以用来将频域图像转换为时域图像,进行图像重建、滤波、增强等操作。
Lower resolution data
本文中,Lower resolution Smaller matrix size 和 Lower effective resolution Same matrix size 是两种不同的降低空间分辨率的方法。前者是通过减少采样矩阵的大小来实现的,后者是通过在原始k空间数据中去除高频分量来实现的。这两种方法的区别在于:
Lower resolution Smaller matrix size 会导致视场变小,因此可能会损失一些感兴趣的脑区的信号。Lower effective resolution Same matrix size 则不会改变视场,而是保留了所有感兴趣的脑区的信号。
Lower resolution Smaller matrix size 会增加噪声水平,因为每个体素包含的信号更少。Lower effective resolution Same matrix size 则不会增加噪声水平,而是减少了高频噪声的影响。
Lower resolution Smaller matrix size 会降低信号的空间频率,因此可能会丢失一些高频信息。Lower effective resolution Same matrix size 也会降低信号的空间频率,但是可以通过重建不同的有效分辨率来控制信息的损失。
BOLD acivation 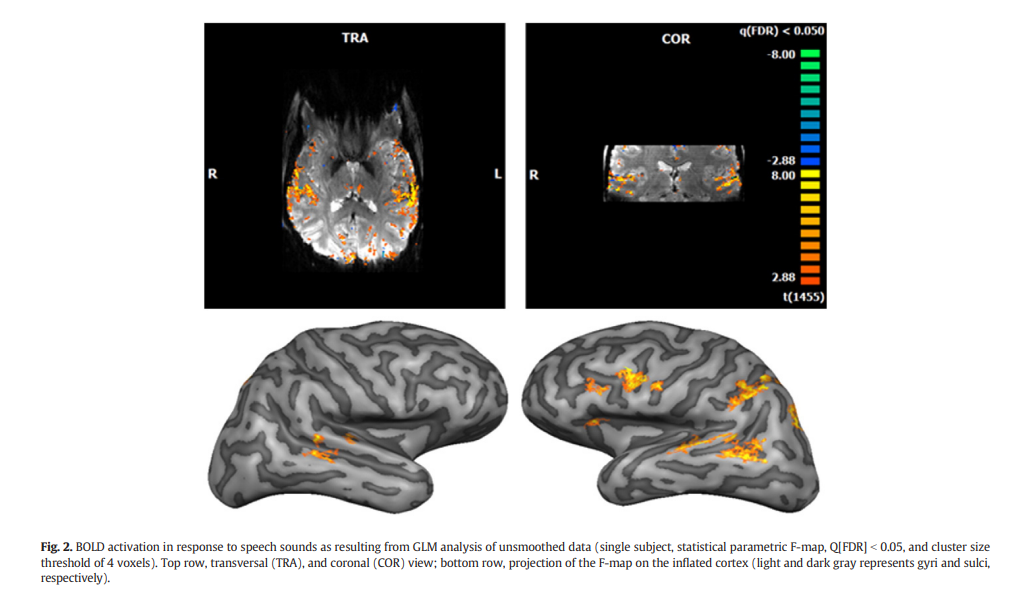
这个图显示了在没有空间平滑的情况下,使用GLM分析单个受试者的fMRI数据得到的BOLD激活图,反映了对语音声音的响应(统计参数F-map,Q[FDR] b 0.05,和簇大小阈值为4个体素)。上面一行是横断面(TRA)和冠状面(COR)的视图;下面一行是将F-map投影到膨胀的皮层上(浅灰色和深灰色分别表示回和沟)。图中的颜色代表了不同的听觉皮层区域,如A1,PT,PP等。这些区域是根据听觉皮层的声学特征和解剖结构来定义的。这个图的目的是展示在高分辨率(1.1 mm)下,fMRI可以捕捉到听觉皮层的细微结构和功能区域。
GLM分析
GLM分析是一种常用的fMRI数据分析方法,它的全称是广义线性模型(General Linear Model)。它是一种基于统计学的方法,用来检测fMRI信号中的任务相关变化。它假设fMRI信号是由不同的成分线性组合而成的,其中包括感兴趣的任务效应、不感兴趣的混杂因素和随机噪声。GLM分析的目的是估计每个成分对fMRI信号的贡献,并进行假设检验,从而推断哪些脑区在任务中被激活。GLM分析是一种单变量分析方法,它只考虑每个体素的fMRI信号,而不考虑不同体素之间的空间模式。本文中,GLM分析被用来与多变量模式分析(MVPA)进行对比,后者是一种利用空间模式信息来解码fMRI数据的方法。
decoding结果
图4.用2.2 mm高斯核平滑后,原始分辨率1.1 mm各向同性数据的组级解码精度。精度被绘制为所选体素的数目。每条线代表所有受试者的平均准确度,误差条代表平均值的标准误差。0.5 附近的细线表示使用乱序标签进行解码的相应结果
声音解码: 这个图显示了用多变量模式分析(MVPA)从听觉皮层的反应模式中解码不同的元音和说话者的准确率。解码是基于支持向量机(SVM)的分类器,它可以根据不同的声音特征区分不同的元音和说话者。
空间平滑: 这个图比较了不同的空间平滑程度对解码准确率的影响。空间平滑是一种图像处理方法,它可以减少噪声和增强信号,但也可能损失一些细节信息。这个图使用了不同大小的高斯核来对原始的1.1毫米等距的数据进行空间平滑,然后选取不同数量的体素进行解码。
不同核空间平滑解码结果对比
这张图显示了:
空间分辨率和平滑对解码性能的影响:图中的每个点表示了在不同的空间分辨率(从后到前:1.1 × 1.1, 2.2 × 2.2, 和 3.3 × 3.3 mm2 平面)和空间平滑核大小(从右到左:无平滑或高斯核大小 1.1, 2.2, 3.3, 4.4, 8.8 mm)下,从听觉皮层反应模式中解码元音(左图)和说话者(右图)的组平均准确率。为了显示的目的,准确率是在所有特征选择水平上的平均结果。
元音和说话者解码的最佳分辨率和平滑:从图中可以看出,对于元音解码,最高的准确率是在 1.1 × 1.1 mm2 分辨率和 2.2 mm 平滑核下达到的(约 0.75)。对于说话者解码,最高的准确率是在 2.2 × 2.2 mm2 分辨率和 2.2 mm 平滑核下达到的(约 0.85)。这表明,不同的解码任务对空间分辨率和平滑的需求是不同的。
空间频率信息的贡献:图中还可以看出,随着分辨率的降低和平滑核的增大,元音解码的准确率呈现出明显的下降趋势,而说话者解码的准确率则相对稳定。这暗示了高空间频率信息对元音解码的重要性,以及高低空间频率信息在两个解码任务中的相对贡献不同。
resolution: resolution 是指 fMRI 数据的空间分辨率,也就是每个体素(voxel)的大小。体素是 fMRI 数据的最小单位,它反映了一个小区域内的神经活动。空间分辨率越高,体素越小,可以捕捉到更多的细节信息,但也可能增加噪声和计算量。空间分辨率越低,体素越大,可以减少噪声和计算量,但也可能损失一些细节信息。本图中使用了三种不同的空间分辨率:1.1 × 1.1, 2.2 × 2.2, 和 3.3 × 3.3 mm2 平面。
smoothing: smoothing 是指对 fMRI 数据进行空间平滑的处理,也就是用一个高斯核(Gaussian kernel)对每个体素周围的体素进行加权平均。空间平滑可以减少噪声和增强信号,但也可能损失一些细节信息。空间平滑的程度取决于高斯核的大小,高斯核越大,平滑程度越高。本图中使用了六种不同的高斯核大小:无平滑或高斯核大小 1.1, 2.2, 3.3, 4.4, 8.8 mm。
四、一篇最新文章
《Global k-Space Interpolation for Dynamic MRI Reconstruction using Masked Image Modeling》2023年
摘要
摘要:在动态磁共振成像(MRI)中,由于扫描时间有限,k空间通常是欠采样的,从而导致图像域中的混叠伪影。因此,动态磁共振重建不仅需要在k空间的x和y方向上对空间频率分量进行建模,还需要考虑时间冗余。以往的工作大多依赖于图像域正则化器(prior)来进行MR重建。相反,我们专注于在用傅里叶变换获得图像之前对欠采样k空间进行插值。在这项工作中,我们将屏蔽图像建模与k空间插值联系起来,并提出了一种新的基于变压器的k空间全局插值网络,称为k-GIN。我们的k-GIN学习2D+t k空间的低频和高频分量之间的全局依赖关系,并使用它来插值未采样数据。此外,我们提出了一种新的k空间迭代细化模块(k-IRM)来增强高频分量的学习。
我们对92名内部2D+t心脏MR受试者评估了我们的方法,并将其与带有图像域正则化器的MR重建方法进行了比较。实验表明,本文提出的k空间插值方法在定量和定性上都优于基线方法。重要的是,所提出的方法在高度欠采样的MR数据中实现了更高的鲁棒性和泛化性。视频演示,海报,GIF结果和代码,请查看我们的项目页面:https://jzpeterpan.github.io/k-gin.github.io/。
主要内容
这篇文章是一篇关于使用遮罩图像建模进行动态MRI重建的全局k空间插值方法的研究论文。它主要介绍了:
- k-GIN网络:一个基于变换器的k空间插值网络,利用遮罩图像建模的原理,从有限的采样数据中学习富有表达力的特征表示,并利用全局依赖关系来估计未采样的k空间数据。
- k-IRM模块:一个k空间迭代细化模块,用于提高k-GIN网络的插值精度,特别是在高频分量上。它使用三个变换器块分别在ky-t,kx-t和kx-ky平面上操作,有效地提取MR数据的时空冗余信息。
- 实验结果:作者在92个内部收集的2D+t心脏MR数据上评估了他们的方法,并与使用图像先验的模型重建方法进行了比较。实验结果表明,他们的方法在定量和定性上都优于基线方法,并且在不同的欠采样因子下具有更好的鲁棒性和泛化性。
主要流程
首先,它使用一个叫做k-GIN的网络,利用遮罩图像建模的技术,来从有限的采样k空间数据中学习全局的特征表示,并用一个解码器来估计未采样的k空间数据。
然后,它使用一个叫做k-IRM的模块,来对k-GIN的估计进行迭代的细化,特别是在高频部分。k-IRM使用三个变换器块,分别在ky-t,kx-t和kx-ky平面上操作,以利用k空间数据的时空冗余。
最后,在推理阶段,它用真实的k空间值替换采样位置的估计值,保证数据一致性。然后,它用傅里叶变换将完整的k空间转换为图像。
missing k-space data是指在采样过程中没有被采集到的k空间数据,它们会导致图像域中出现混叠伪影。因此,重建的目标是从已采样的k空间数据中插值出missing k-space data,从而得到无伪影的图像。
这个流程图是用来说明k-IRM的工作原理的。它显示了k-IRM如何利用三个不同的平面(ky-t, kx-t, kx-ky)来提高k-空间数据的高频分量的精度。每个平面都有一个Transformer块,可以捕捉k-空间数据的时空冗余,并迭代地减少重建误差。最后,k-IRM的输出与k-GIN的输出相结合,得到完整的k-空间数据,然后通过傅里叶变换得到图像重建。
(ky-t, kx-t, kx-ky)是本文中用来描述k-space数据的三个正交平面的简称。k-space数据是MR成像的频域数据,可以看作是一个三维矩阵,其中kx, ky, t分别表示水平、垂直和时间维度。本文的方法是在这三个平面上分别使用Transformer网络来提取k-space数据的全局依赖关系,并利用这些信息来插补未采样的k-space数据。具体来说:
ky-t平面是将kx维度视为通道维度,将ky和t维度视为空间维度的平面。在这个平面上,每个点都是一个token,可以用来学习k-space数据在ky和t方向上的冗余。
kx-t平面是将ky维度视为通道维度,将kx和t维度视为空间维度的平面。在这个平面上,每个点也是一个token,可以用来学习k-space数据在kx和t方向上的冗余。
kx-ky平面是将t维度视为通道维度,将kx和ky维度视为空间维度的平面。在这个平面上,每个点是一个4×4的小块,可以用来学习k-space数据在kx和ky方向上的冗余。
原文地址:https://blog.csdn.net/qq_24211837/article/details/134728100
本文来自互联网用户投稿,该文观点仅代表作者本人,不代表本站立场。本站仅提供信息存储空间服务,不拥有所有权,不承担相关法律责任。
如若转载,请注明出处:http://www.7code.cn/show_25454.html
如若内容造成侵权/违法违规/事实不符,请联系代码007邮箱:suwngjj01@126.com进行投诉反馈,一经查实,立即删除!